class: center, middle, inverse, title-slide #
Linear Models and Clustered Data
## Data Analysis for Psychology in R 3 ### Josiah King, Umberto Noè, Tom Booth ### Department of Psychology
The University of Edinburgh ### AY 2021-2022 --- --- class: inverse, center, middle <h2>Part 1: Linear Regression Refresh</h2> <h2 style="text-align: left;opacity:0.3;">Part 2: Clustered Data</h2> <h2 style="text-align: left;opacity:0.3;">Part 3: Possible Approaches</h2> <h2 style="text-align: left;opacity:0.3;">Extra: ANOVA & Repeated Measures (brief)</h2> --- # Models .pull-left[ __deterministic__ given the same input, deterministic functions return *exactly* the same output - `\(y = mx + c\)` - area of sphere = `\(4\pi r^2\)` - height of fall = `\(1/2 g t^2\)` - `\(g = \textrm{gravitational constant, }9.8m/s^2\)` - `\(t = \textrm{time (in seconds) of fall}\)` ] -- .pull-right[ __statistical__ .br2.f4.white.bg-gray[ $$ \textrm{outcome} = (\textrm{model}) + \color{black}{\textrm{error}} $$ ] - handspan = height + randomness - cognitive test score = age + premorbid IQ + ... + randomness ] --- # The Linear Model .br3.pa2.f2[ $$ `\begin{align} \color{red}{\textrm{outcome}} & = \color{blue}{(\textrm{model})} + \textrm{error} \\ \color{red}{y_i} & = \color{blue}{\beta_0 \cdot{} 1 + \beta_1 \cdot{} x_i} + \varepsilon_i \\ \text{where } \\ \varepsilon_i & \sim N(0, \sigma) \text{ independently} \\ \end{align}` $$ ] --- # Model structure .flex.items-top[ .w-50.pa2[ Our proposed model of the world: `\(\color{red}{y_i} = \color{blue}{\beta_0 \cdot{} 1 + \beta_1 \cdot{} x_i} + \varepsilon_i\)` {{content}} ] .w-50.pa2[ 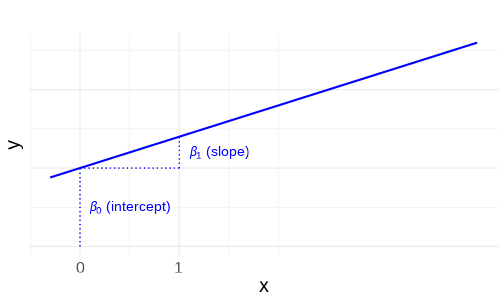<!-- --> ]] -- Our model _fitted_ to some data (note the `\(\widehat{\textrm{hats}}\)`): `\(\hat{y}_i = \color{blue}{\hat \beta_0 \cdot{} 1 + \hat \beta_1 \cdot{} x_i}\)` {{content}} -- For the `\(i^{th}\)` observation: - `\(\color{red}{y_i}\)` is the value we observe for `\(x_i\)` - `\(\hat{y}_i\)` is the value the model _predicts_ for `\(x_i\)` - `\(\color{red}{y_i} = \hat{y}_i + \varepsilon_i\)` --- # An Example .flex.items-top[ .w-50.pa2[ `\(\color{red}{y_i} = \color{blue}{5 \cdot{} 1 + 2 \cdot{} x_i} + \varepsilon_i\)` {{content}} ] .w-50.pa2[ 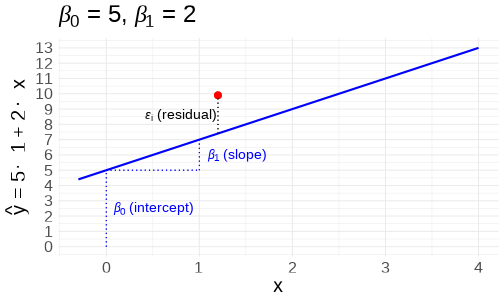<!-- --> ]] -- __e.g.__ for the observation `\(x_i = 1.2, \; y_i = 9.9\)`: $$ `\begin{align} \color{red}{9.9} & = \color{blue}{5 \cdot{}} 1 + \color{blue}{2 \cdot{}} 1.2 + \varepsilon_i \\ & = 7.4 + \varepsilon_i \\ & = 7.4 + 2.5 \\ \end{align}` $$ --- # Extending the linear model ## Categorical Predictors .pull-left[ <br><table> <thead> <tr> <th style="text-align:left;"> y </th> <th style="text-align:left;"> x </th> </tr> </thead> <tbody> <tr> <td style="text-align:left;"> 7.99 </td> <td style="text-align:left;"> Category1 </td> </tr> <tr> <td style="text-align:left;"> 4.73 </td> <td style="text-align:left;"> Category0 </td> </tr> <tr> <td style="text-align:left;"> 3.66 </td> <td style="text-align:left;"> Category0 </td> </tr> <tr> <td style="text-align:left;"> 3.41 </td> <td style="text-align:left;"> Category0 </td> </tr> <tr> <td style="text-align:left;"> 5.75 </td> <td style="text-align:left;"> Category1 </td> </tr> <tr> <td style="text-align:left;"> 5.66 </td> <td style="text-align:left;"> Category0 </td> </tr> <tr> <td style="text-align:left;"> ... </td> <td style="text-align:left;"> ... </td> </tr> </tbody> </table> ] .pull-right[ 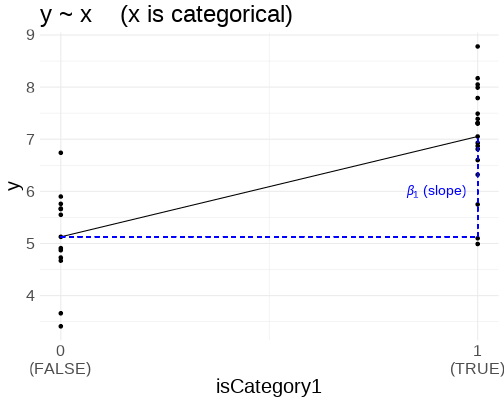<!-- --> ] --- # Extending the linear model ## Multiple predictors .pull-left[ 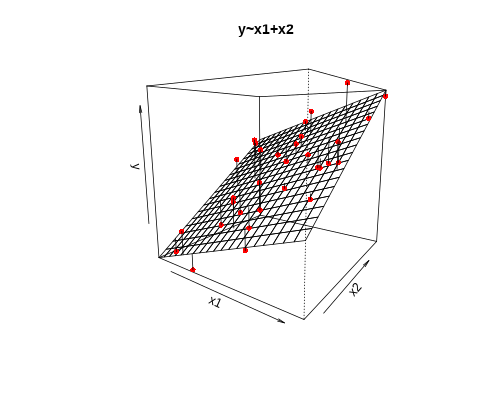<!-- --> ] -- .pull-right[ 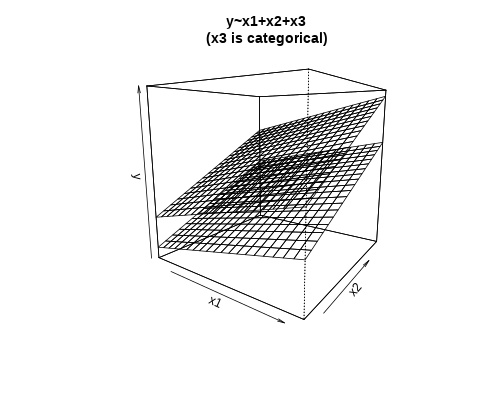<!-- --> ] --- # Extending the linear model ## Interactions .pull-left[ 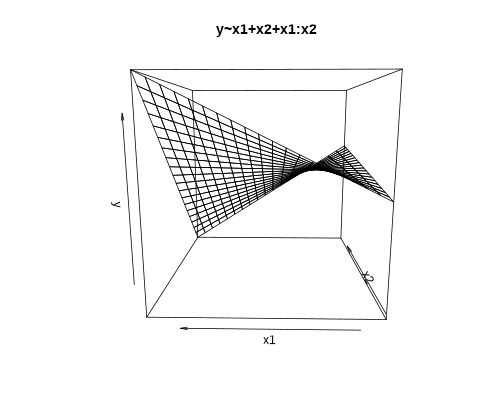<!-- --> ] -- .pull-right[ 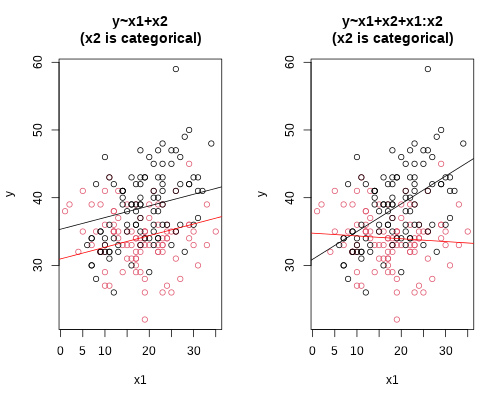<!-- --> ] --- # Notation `\(\begin{align} \color{red}{y} \;\;\;\; & = \;\;\;\;\; \color{blue}{\beta_0 \cdot{} 1 + \beta_1 \cdot{} x_1 + ... + \beta_k \cdot x_k} & + & \;\;\;\varepsilon \\ \qquad \\ \color{red}{\begin{bmatrix}y_1 \\ y_2 \\ y_3 \\ y_4 \\ y_5 \\ \vdots \\ y_n \end{bmatrix}} & = \color{blue}{\begin{bmatrix} 1 & x_{11} & x_{21} & \dots & x_{k1} \\ 1 & x_{12} & x_{22} & & x_{k2} \\ 1 & x_{13} & x_{23} & & x_{k3} \\ 1 & x_{14} & x_{24} & & x_{k4} \\ 1 & x_{15} & x_{25} & & x_{k5} \\ \vdots & \vdots & \vdots & \ddots & \vdots \\ 1 & x_{1n} & x_{2n} & \dots & x_{kn} \end{bmatrix} \begin{bmatrix} \beta_0 \\ \beta_1 \\ \beta_2 \\ \vdots \\ \beta_k \end{bmatrix}} & + & \begin{bmatrix} \varepsilon_1 \\ \varepsilon_2 \\ \varepsilon_3 \\ \varepsilon_4 \\ \varepsilon_5 \\ \vdots \\ \varepsilon_n \end{bmatrix} \\ \qquad \\ \\\color{red}{\boldsymbol y} \;\;\;\;\; & = \qquad \qquad \;\;\; \mathbf{\color{blue}{X \qquad \qquad \qquad \;\;\;\: \boldsymbol \beta}} & + & \;\;\; \boldsymbol \varepsilon \\ \end{align}\)` --- # Extending the linear model ## Link functions $$ `\begin{align} \color{red}{y} = \mathbf{\color{blue}{X \boldsymbol{\beta}} + \boldsymbol{\varepsilon}} & \qquad & (-\infty, \infty) \\ \qquad \\ \qquad \\ \color{red}{ln \left( \frac{p}{1-p} \right) } = \mathbf{\color{blue}{X \boldsymbol{\beta}} + \boldsymbol{\varepsilon}} & \qquad & [0,1] \\ \qquad \\ \qquad \\ \color{red}{ln (y) } = \mathbf{\color{blue}{X \boldsymbol{\beta}} + \boldsymbol{\varepsilon}} & \qquad & (0, \infty) \\ \end{align}` $$ --- # Linear Models in R - Linear regression ```r linear_model <- lm(continuous_y ~ x1 + x2 + x3*x4, data = df) ``` - Logistic regression ```r logistic_model <- glm(binary_y ~ x1 + x2 + x3*x4, data = df, family=binomial(link="logit")) ``` - Poisson regression ```r poisson_model <- glm(count_y ~ x1 + x2 + x3*x4, data = df, family=poisson(link="log")) ``` --- # Inference for the linear model <img src="jk_img_sandbox/sum1.png" width="2560" height="500px" /> --- # Inference for the linear model <img src="jk_img_sandbox/sum2.png" width="2560" height="500px" /> --- # Inference for the linear model <img src="jk_img_sandbox/sum3.png" width="2560" height="500px" /> --- # Inference for the linear model <img src="jk_img_sandbox/sum4.png" width="2560" height="500px" /> --- # Assumptions Our model: `\(\color{red}{y} = \color{blue}{\mathbf{X \boldsymbol \beta}} + \varepsilon \\ \text{where } \boldsymbol \varepsilon \sim N(0, \sigma) \text{ independently}\)` -- Our ability to generalise from the model we fit on sample data to the wider population requires making some _assumptions._ -- - assumptions about the nature of the **model** .tr[ (linear) ] -- - assumptions about the nature of the **errors** .tr[ (normal) ] -- .footnote[ You can also phrase the linear model as: `\(\color{red}{\boldsymbol y} \sim Normal(\color{blue}{\mathbf{X \boldsymbol \beta}}, \sigma)\)` ] --- # The Broad Idea All our work here is in aim of making **models of the world**. -- - Models are models. They are simplifications. They are therefore wrong. -- 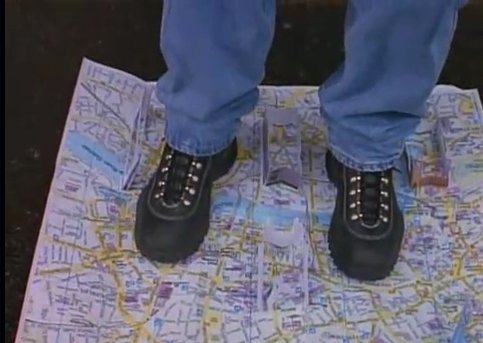 --- count:false # The Broad Idea All our work here is in aim of making **models of the world**. - Models are models. They are simplifications. They are therefore wrong. - Our residuals ( `\(y - \hat{y}\)` ) reflect everything that we **don't** account for in our model. -- - In an ideal world, our model accounts for _all_ the systematic relationships. The leftovers (our residuals) are just random noise. -- - If our model is mis-specified, or we don't measure some systematic relationship, then our residuals will reflect this. -- - We check by examining how much "like randomness" the residuals appear to be (zero mean, normally distributed, constant variance, i.i.d ("independent and identically distributed") - _these ideas tend to get referred to as our "assumptions"_ -- - We will **never** know whether our residuals contain only randomness - we can never observe everything! --- # Checking Assumptions .pull-left[ What does "zero mean and constant variance" look like? - mean of the residuals = zero across the predicted values on the linear predictor. - spread of residuals is normally distributed and constant across the predicted values on the linear predictor. ] .pull-right[ 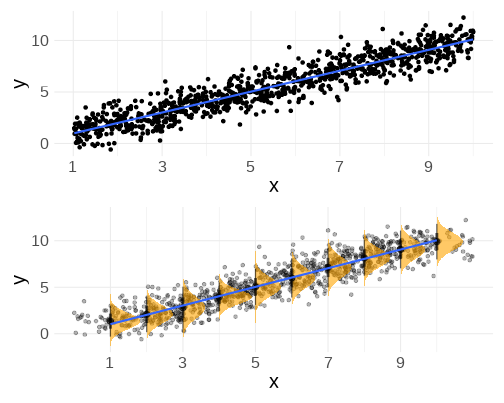<!-- --> ] --- count:false # Checking Assumptions .pull-left[ What does "zero mean and constant variance" look like? - __mean of the residuals = zero across the predicted values on the linear predictor.__ - spread of residuals is normally distributed and constant across the predicted values on the linear predictor. ] .pull-right[ 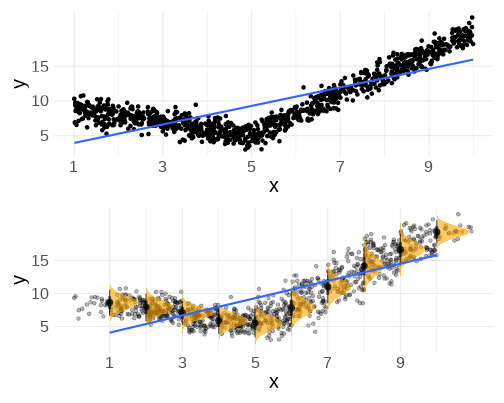<!-- --> ] --- count:false # Checking Assumptions .pull-left[ What does "zero mean and constant variance" look like? - mean of the residuals = zero across the predicted values on the linear predictor. - __spread of residuals is normally distributed and constant across the predicted values on the linear predictor.__ ] .pull-right[ 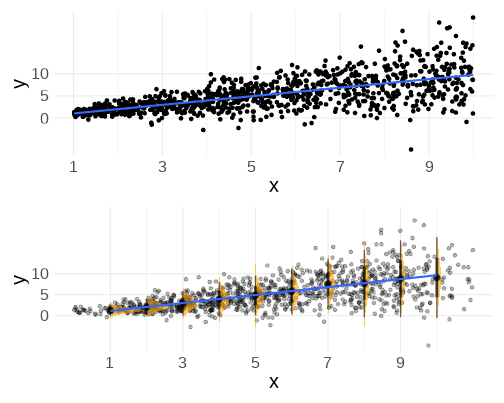<!-- --> ] --- count:false # Checking Assumptions .pull-left[ What does "zero mean and constant variance" look like? - mean of the residuals = zero across the predicted values on the linear predictor. - spread of residuals is normally distributed and constant across the predicted values on the linear predictor. ] .pull-right[ __`plot(model)`__ ```r my_model <- lm(y ~ x, data = df) plot(my_model, which = 1) ``` 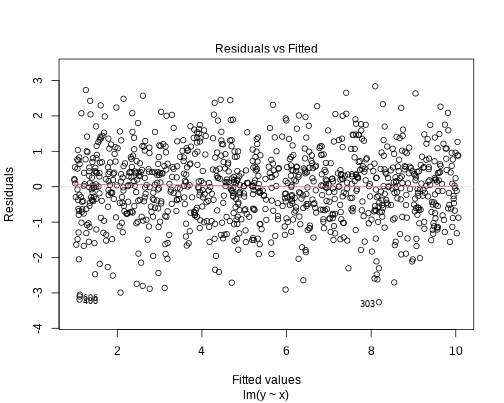<!-- --> ] --- count:false # Checking Assumptions <br><br> <center> <div class="acronym"> L </div> inearity<br> <div class="acronym"> I </div> ndependence<br> <div class="acronym"> N </div> ormality<br> <div class="acronym"> E </div> qual variance<br> </center> .footnote["Line without N is a Lie!" (Umberto)] --- # What if our model doesn't meet assumptions? - is our model mis-specified? - is the relationship non-linear? higher order terms? (e.g. `\(y \sim x + x^2\)`) - is there an omitted variable or interaction term? -- - transform the outcome variable? - makes things look more "normal" - but can make things more tricky to interpret: `\(y \sim x\)` and `\(log(y) \sim x\)` are quite different models -- - bootstrap - do many times: resample (w/ replacement) your data, and refit your model. - obtain a distribution of parameter estimate of interest. - compute a confidence interval for estimate - celebrate -- But these don't help if we have violated our assumption of independence... --- # Summary - we can fit a linear regression model which takes the form `\(\color{red}{y} = \color{blue}{\mathbf{X} \boldsymbol{\beta}} + \boldsymbol{\varepsilon}\)` -- - in R, we fit this with `lm(y ~ x1 + .... xk, data = mydata)`. -- - we can extend this to different link functions to model outcome variables which follow different distributions. -- - when drawing inferences from a fitted model to the broader population, we rely on certain assumptions. -- - one of these is that the errors are independent. --- class: inverse, center, middle, animated, rotateInDownLeft # End of Part 1 --- class: inverse, center, middle <h2 style="text-align: left;opacity:0.3;">Part 1: Linear Regression Refresh</h2> <h2>Part 2: Clustered Data</h2> <h2 style="text-align: left;opacity:0.3;">Part 3: Possible Approaches</h2> <h2 style="text-align: left;opacity:0.3;">Extra: ANOVA & Repeated Measures (brief)</h2> --- # What is clustered data? .pull-left[ - children within schools - patients within clinics - observations within individuals ] .pull-left[ <img src="jk_img_sandbox/h2.png" width="1716" /> ] --- count:false # What is clustered data? .pull-left[ - children within classrooms within schools within districts etc... - patients within doctors within hospitals... - time-periods within trials within individuals ] .pull-right[ <img src="jk_img_sandbox/h3.png" width="1716" /> ] -- .footnote[ Other relevant terms you will tend to see: "grouping structure", "levels", "hierarchies". ] --- # Why is clustering worth thinking about? Clustering will likely result in measurements on observational units within a given cluster being more similar to each other than to those in other clusters. -- <br> - For example, our measure of academic performance for children in a given class will tend to be more similar to one another (because of class specific things such as the teacher) than to children in other classes. -- .pull-left[ A lot of the data you will come across will have clusters. - multiple experimental trials per participant - patients in clinics - employees within departments - children within classes ] -- .pull-right[ <img src="jk_img_sandbox/lev1.png" width="1657" /> ] --- # ICC (intra-class correlation coefficient) Clustering is expressed in terms of the correlation among the measurements within the same cluster - known as the __intra-class correlation coefficient (ICC).__ -- There are various formulations of ICC, but the basic principle = ratio of *variance between groups* to *total variance*. <br> `\(\rho = \frac{\sigma^2_{b}}{\sigma^2_{b} + \sigma^2_e} \\ \qquad \\\textrm{Where:} \\ \sigma^2_{b} = \textrm{variance between clusters} \\ \sigma^2_e = \textrm{variance within clusters (residual variance)} \\\)` -- Can also be interpreted as the correlation between two observations from the same group. --- # Various values of `\(\rho\)` The larger the ICC, the lower the variability is within the clusters (relative to the variability between clusters). The greater the correlation between two observations from the same group. .pull-left[ <img src="01_lmcluster_files/figure-html/unnamed-chunk-22-1.png" style="display: block; margin: auto;" /> ] -- .pull-right[ <img src="01_lmcluster_files/figure-html/unnamed-chunk-23-1.png" style="display: block; margin: auto;" /> ] --- # Clustered data & lm .pull-left[ #### Why is it a problem? Clustering is something **systematic** that our model should (arguably) take into account. - remember, `\(\varepsilon \sim N(0, \sigma) \textbf{ independently}\)` ] -- .pull-right[ #### HOW is it a problem? Standard errors tend to be smaller than they should be, meaning that: - confidence intervals will be too narrow - `\(t\)`-statistics will be too large - `\(p\)`-values will be misleadingly small ] --- class: inverse, center, middle <h2 style="text-align: left;opacity:0.3;">Part 1: Linear Regression Refresh</h2> <h2><b style="opacity:0.4;">Part 2: Clustered Data</b><b>A Practical Comment on Data</b></h2> <h2 style="text-align: left;opacity:0.3;">Part 3: Possible Approaches</h2> <h2 style="text-align: left;opacity:0.3;">Extra: ANOVA & Repeated Measures (brief)</h2> --- # Wide Data/Long Data .pull-left[ __Wide Data__ ``` ## # A tibble: 5 × 5 ## ID age trial_1 trial_2 trial_3 ## <chr> <chr> <chr> <chr> <chr> ## 1 001 28 10 12.5 18 ## 2 002 36 7.5 7 5 ## 3 003 61 12 14.5 11 ## 4 004 45 10.5 17 14 ## 5 ... ... ... ... ... ``` ] .pull-right[ __Long Data__ ``` ## # A tibble: 13 × 4 ## ID age trial score ## <chr> <chr> <chr> <chr> ## 1 001 36 trial_1 10 ## 2 001 36 trial_2 12.5 ## 3 001 36 trial_3 18 ## 4 002 70 trial_1 7.5 ## 5 002 70 trial_2 7 ## 6 002 70 trial_3 5 ## 7 003 68 trial_1 12 ## 8 003 68 trial_2 14.5 ## 9 003 68 trial_3 11 ## 10 004 31 trial_1 10.5 ## 11 004 31 trial_2 17 ## 12 004 31 trial_3 14 ## 13 ... ... ... ... ``` ] --- # Wide Data/Long Data ](https://www.fromthebottomoftheheap.net/assets/img/posts/tidyr-longer-wider.gif) --- # Long is good for plotting. .pull-left[ __`group` aesthetic__ ```r ggplot(longd, aes(x=trial,y=score, group=ID, col=ID))+ geom_point(size=4)+ geom_path()+ themedapr3() ``` 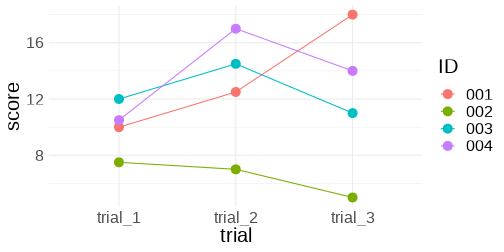<!-- --> ] .pull-right[ __`facet_wrap()`__ ```r ggplot(longd, aes(x=trial,y=score))+ geom_point(size=4)+ geom_path(aes(group=1))+ themedapr3()+ facet_wrap(~ID) ``` 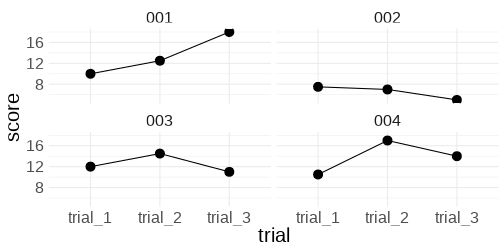<!-- --> ] --- # Long is good for describing by ID ```r longd %>% group_by(ID) %>% summarise( ntrials = n_distinct(trial), meanscore = mean(score), sdscore = sd(score) ) ``` ``` ## # A tibble: 4 × 4 ## ID ntrials meanscore sdscore ## <chr> <int> <dbl> <dbl> ## 1 001 3 13.5 4.09 ## 2 002 3 6.5 1.32 ## 3 003 3 12.5 1.80 ## 4 004 3 13.8 3.25 ``` --- # Summary - Clustering can take many forms, and exist at many levels -- - Clustering is something systematic that we would want our model to take into account - Ignoring it can lead to incorrect statistical inferences -- - Clustering is typically assessed using intra-class correlation coefficient (ICC) - the ratio of variance between clusters to the total variance `\(\rho = \frac{\sigma^2_b}{\sigma^2_b + \sigma^2_e}\)` -- - Tidying your data and converting it to *long* format (one observational unit per row, and a variable identifying the cluster ID) is a good start. --- class: inverse, center, middle, animated, rotateInDownLeft # End of Part 2 --- class: inverse, center, middle <h2 style="text-align: left;opacity:0.3;">Part 1: Linear Regression Refresh</h2> <h2 style="text-align: left;opacity:0.3;">Part 2: Clustered Data</h2> <h2>Part 3: Possible Approaches</h2> <h2 style="text-align: left;opacity:0.3;">Extra: ANOVA & Repeated Measures (brief)</h2> --- # Our Data .pull-left[ > Sample of 115 birds from 12 gardens, information captured on the arrival time (hours past midnight) and number of worms caught by the end of the day. ```r worms_data <- read_csv("https://uoepsy.github.io/data/worms.csv") head(worms_data) ``` ``` ## # A tibble: 6 × 5 ## gardenid birdid arrivalt nworms birdt ## <chr> <dbl> <dbl> <dbl> <chr> ## 1 garden1 1 6.49 53 blackbird ## 2 garden1 2 6.32 54 blackbird ## 3 garden1 3 7.15 52 blackbird ## 4 garden1 4 6.03 51 wren ## 5 garden1 5 6.38 51 wren ## 6 garden1 6 5.47 55 blackbird ``` ```r library(ICC) ICCbare(x = gardenid, y = nworms, data = worms_data) ``` ``` ## [1] 0.9549 ``` ] .pull-right[ <img src="01_lmcluster_files/figure-html/unnamed-chunk-32-1.png" style="display: block; margin: auto;" /> ] --- # Ignore the clustering .pull-left[ __(Complete pooling)__ + `lm(y ~ 1 + x, data = df)` + Information from all clusters is pooled together to estimate over x ```r model <- lm(nworms ~ arrivalt, data = worms_data) ``` ``` ## Estimate Std. Error t value Pr(>|t|) ## (Intercept) 18.08 8.68 2.08 0.040 * ## arrivalt 3.29 1.31 2.50 0.014 * ``` ] .pull-right[ 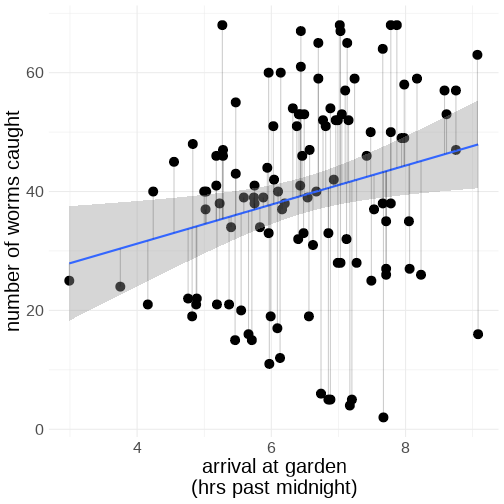<!-- --> ] --- count:false # Ignore the clustering .pull-left[ __(Complete pooling)__ + `lm(y ~ 1 + x, data = df)` + Information from all clusters is pooled together to estimate over x ```r model <- lm(nworms ~ arrivalt, data = worms_data) ``` ``` ## Estimate Std. Error t value Pr(>|t|) ## (Intercept) 18.08 8.68 2.08 0.040 * ## arrivalt 3.29 1.31 2.50 0.014 * ``` But different clusters show different patterns. Residuals are not independent. ] .pull-right[ 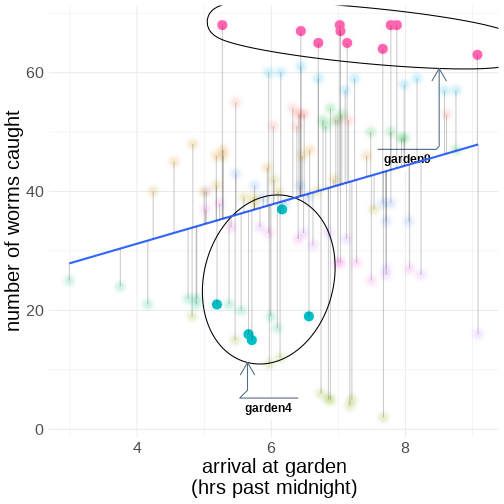<!-- --> ] --- count:false class: extra exclude: FALSE # Lesser used approaches __Cluster Robust Standard Errors__ .pull-left[ <!-- https://rlbarter.github.io/Practical-Statistics/2017/05/10/generalized-estimating-equations-gee/ --> Don't include clustering as part of the model directly, but incorporate the dependency into our residuals term. $$ `\begin{align} \color{red}{\textrm{outcome}} & = \color{blue}{(\textrm{model})} + \textrm{error}^* \\ \end{align}` $$ .footnote[`*` Where errors are clustered] ] -- .pull-right[ Simply adjusts our standard errors: ```r library(plm) clm <- plm(nworms ~ 1 + arrivalt, data=worms_data, model="pooling", index="gardenid") ``` ``` ## Coefficients: ## Estimate Std. Error t-value Pr(>|t|) ## (Intercept) 18.08 8.68 2.08 0.040 * ## arrivalt 3.29 1.31 2.50 0.014 * ``` ```r sqrt(diag(vcovHC(clm, method='arellano', cluster='group'))) ``` ``` ## (Intercept) arrivalt ## 12.890 2.138 ``` ] --- class: extra exclude: FALSE count:false # Lesser used approaches __Generalised Estimating Equations (GEE)__ .pull-left[ - Don't include clustering as part of the model directly, but incorporate the dependency into our residuals term. $$ `\begin{align} \color{red}{\textrm{outcome}} & = \color{blue}{(\textrm{model})} + \textrm{error}^* \\ \end{align}` $$ .footnote[`*` Where errors are clustered, and follow some form of correlational<br>structure within clusters (e.g. based on how many timepoints apart<br>two observations are).] ] -- .pull-right[ Specifying a correlational structure for residuals within clusters can influence _what_ we are estimating ```r library(geepack) # needs to be arranged by cluster, # and for cluster to be numeric worms_data <- worms_data %>% mutate( cluster_id = as.numeric(as.factor(gardenid)) ) %>% arrange(cluster_id) # geemod = geeglm(nworms ~ 1 + arrivalt, data = worms_data, corstr = 'exchangeable', id = cluster_id) ``` ``` ## Coefficients: ## Estimate Std.err Wald Pr(>|W|) ## (Intercept) 54.137 4.344 155.3 < 2e-16 *** ## arrivalt -2.384 0.619 14.8 0.00012 *** ``` ] --- # Fixed effects .pull-left[ __(No pooling)__ - `lm(y ~ x * cluster, data = df)` - Information from a cluster contributes to estimate *for that cluster*, but information is not pooled to estimate an overall effect. ```r model <- lm(nworms ~ 1 + arrivalt * gardenid, data = worms_data) ``` {{content}} ] .pull-right[ 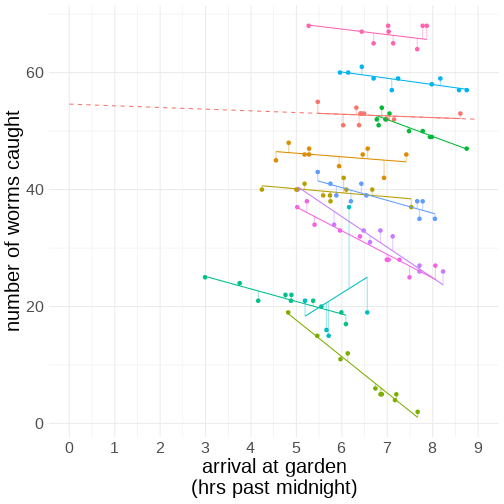<!-- --> ] -- + Lots of estimates (separate for each cluster). + Variance estimates constructed based on information *only* within each cluster. + No overall estimate of effect over x. ``` ## Estimate Std. Error t value Pr(>|t|) ## (Intercept) 54.613 6.032 9.05 2.4e-14 *** ## arrivalt -0.289 0.904 -0.32 0.7502 ## gardenidgarden10 -5.342 7.608 -0.70 0.4844 ## gardenidgarden11 -11.088 7.753 -1.43 0.1561 ## gardenidgarden12 -5.843 8.247 -0.71 0.4804 ## gardenidgarden2 17.571 10.464 1.68 0.0966 . ## gardenidgarden3 -23.058 7.118 -3.24 0.0017 ** ## gardenidgarden4 -61.517 14.101 -4.36 3.4e-05 *** ## gardenidgarden5 11.945 8.158 1.46 0.1466 ## gardenidgarden6 -1.198 8.057 -0.15 0.8821 ## gardenidgarden7 12.145 7.577 1.60 0.1124 ## gardenidgarden8 2.617 7.671 0.34 0.7338 ## gardenidgarden9 18.480 8.130 2.27 0.0254 * ## arrivalt:gardenidgarden10 -0.323 1.196 -0.27 0.7881 ## arrivalt:gardenidgarden11 -0.390 1.229 -0.32 0.7517 ## arrivalt:gardenidgarden12 -5.933 1.247 -4.76 7.3e-06 *** ## arrivalt:gardenidgarden2 -2.599 1.459 -1.78 0.0782 . ## arrivalt:gardenidgarden3 -1.850 1.185 -1.56 0.1219 ## arrivalt:gardenidgarden4 5.156 2.350 2.19 0.0308 * ## arrivalt:gardenidgarden5 -0.787 1.171 -0.67 0.5035 ## arrivalt:gardenidgarden6 -1.892 1.197 -1.58 0.1173 ## arrivalt:gardenidgarden7 -4.942 1.108 -4.46 2.3e-05 *** ## arrivalt:gardenidgarden8 -3.756 1.157 -3.25 0.0016 ** ## arrivalt:gardenidgarden9 -0.655 1.175 -0.56 0.5784 ``` --- # Random effects (MLM) .pull-left[ __(Partial Pooling)__ - `lmer(y ~ 1 + x + (1 + x| cluster), data = df)` - cluster-level variance in intercepts and slopes is modeled as randomly distributed around fixed parameters. - effects are free to vary by cluster, but information from clusters contributes (according to cluster `\(n\)` and outlyingness of cluster) to an overall fixed parameter. ```r library(lme4) model <- lmer(nworms ~ 1 + arrivalt + (1 + arrivalt | gardenid), data = worms_data) summary(model)$coefficients ``` ``` ## Estimate Std. Error t value ## (Intercept) 52.5 4.957 10.59 ## arrivalt -2.1 0.644 -3.26 ``` ] .pull-right[ 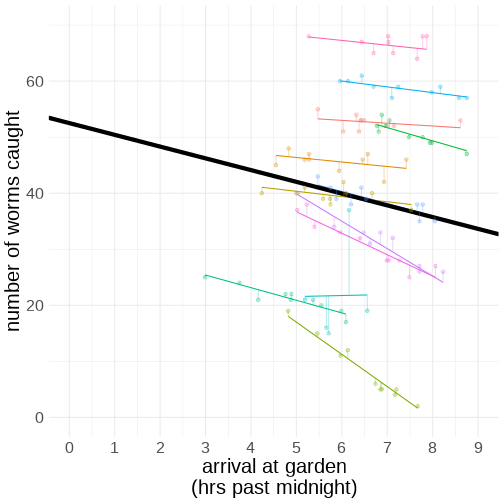<!-- --> ] --- count:false # Random effects (MLM) .pull-left[ __(Partial Pooling)__ - `lmer(y ~ 1 + x + (1 + x| cluster), data = df)` - cluster-level variance in intercepts and slopes is modeled as randomly distributed around fixed parameters. - effects are free to vary by cluster, but information from clusters contributes (according to cluster `\(n\)` and outlyingness of cluster) to an overall fixed parameter. ```r library(lme4) model <- lmer(nworms ~ 1 + arrivalt + (1 + arrivalt | gardenid), data = worms_data) summary(model)$coefficients ``` ``` ## Estimate Std. Error t value ## (Intercept) 52.5 4.957 10.59 ## arrivalt -2.1 0.644 -3.26 ``` ] .pull-right[ 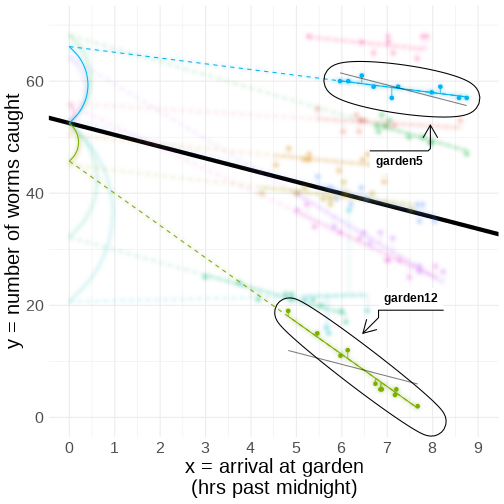<!-- --> ] --- # Summary With clustered data, there are many possible approaches. Some of the main ones are: - Ignore it (**complete pooling**) - and make inappropriate inferences. -- - Completely partition out any variance due to clustering into fixed effects for each cluster (__no pooling__). - and limit our estimates to being cluster specific and low power. -- - Model cluster-level variance as randomly distributed around fixed parameters, and partially pool information across clusters. - best of both worlds? --- class: inverse, center, middle, animated, rotateInDownLeft # End --- exclude: FALSE class: extra, inverse, center, middle <h2 style="text-align: left;opacity:0.3;">Part 1: Linear Regression Refresh</h2> <h2 style="text-align: left;opacity:0.3;">Part 2: Clustered Data</h2> <h2 style="text-align: left;opacity:0.3;">Part 3: Possible Approaches</h2> <h2>Extra: ANOVA & Repeated Measures (brief)</h2> --- count:false class: extra exclude: FALSE # ANOVA revisited - ANOVA in it's simplest form is essentially a linear model with categorical predictor(s). It is used to examines differences in group means/differences in differences in group means etc. - It achieves this by partitioning variance. We can calculate it by hand! - Still quite popular in psychology because we often design experiments with discrete conditions. - This means we can balance designs and examine mean differences between conditions. --- count:false class: extra exclude: FALSE # quick simulating data .pull-left[ ```r testdata <- tibble( groups = rep(c("A","B"), each = 30), outcome = c(rnorm(30,10,5), rnorm(30,15,5)) ) ``` ].pull-right[ ```r with(testdata, boxplot(outcome ~ groups)) ``` 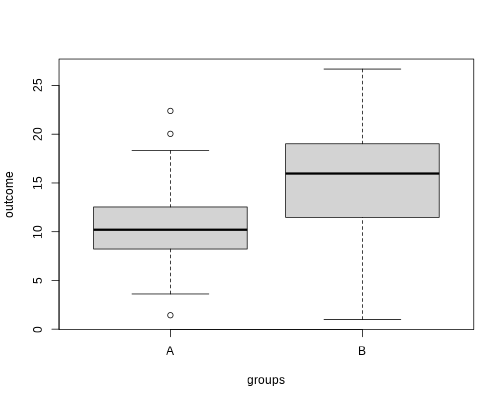<!-- --> ] --- count:false class: extra exclude: FALSE # lm vs anova .pull-left[ ```r summary(lm(outcome ~ groups, data = testdata)) ``` ``` ## ## Call: ## lm(formula = outcome ~ groups, data = testdata) ## ## Residuals: ## Min 1Q Median 3Q Max ## -14.439 -2.614 -0.076 3.141 11.654 ## ## Coefficients: ## Estimate Std. Error t value Pr(>|t|) ## (Intercept) 10.735 0.939 11.43 < 2e-16 *** ## groupsB 4.693 1.329 3.53 0.00082 *** ## --- ## Signif. codes: 0 '***' 0.001 '**' 0.01 '*' 0.05 '.' 0.1 ' ' 1 ## ## Residual standard error: 5.15 on 58 degrees of freedom ## Multiple R-squared: 0.177, Adjusted R-squared: 0.163 ## F-statistic: 12.5 on 1 and 58 DF, p-value: 0.000816 ``` ] .pull-right[ ```r summary(aov(outcome ~ groups, data = testdata)) ``` ``` ## Df Sum Sq Mean Sq F value Pr(>F) ## groups 1 330 330 12.5 0.00082 *** ## Residuals 58 1535 26 ## --- ## Signif. codes: 0 '***' 0.001 '**' 0.01 '*' 0.05 '.' 0.1 ' ' 1 ``` ```r anova(lm(outcome ~ groups, data = testdata)) ``` ``` ## Analysis of Variance Table ## ## Response: outcome ## Df Sum Sq Mean Sq F value Pr(>F) ## groups 1 330 330 12.5 0.00082 *** ## Residuals 58 1535 26 ## --- ## Signif. codes: 0 '***' 0.001 '**' 0.01 '*' 0.05 '.' 0.1 ' ' 1 ``` ] in this case (one predictor) the coefficient test `\(t = \sqrt{F}\)` ```r sqrt(12.5) ``` ``` ## [1] 3.54 ``` --- count:false class: extra exclude: FALSE # more anova - ANOVA tests whether several parameters (differences between group means) are simultaneously zero. - This is why it is referred to as the "omnibus"/"overall F" test. .pull-left[ 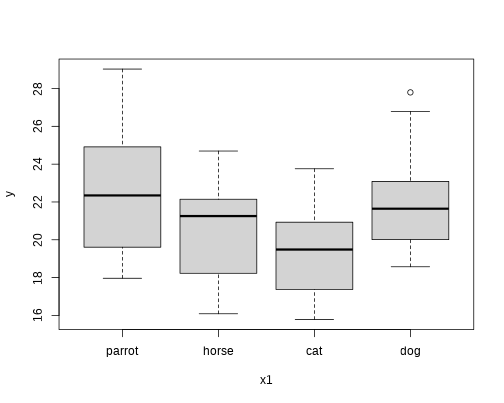<!-- --> ] .pull-right[ ```r anova(lm(y~x1,df)) ``` ``` ## Analysis of Variance Table ## ## Response: y ## Df Sum Sq Mean Sq F value Pr(>F) *## x1 3 67.1 22.37 2.65 0.063 . ## Residuals 36 303.6 8.43 ## --- ## Signif. codes: 0 '***' 0.001 '**' 0.01 '*' 0.05 '.' 0.1 ' ' 1 ``` ] --- count:false class: extra exclude: FALSE # more anova - ANOVA tests whether several parameters (differences between group means) are simultaneously zero. - This is why it is referred to as the "omnibus"/"overall F" test. .pull-left[ 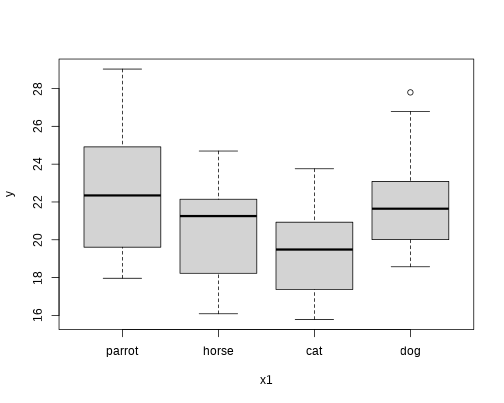<!-- --> ] .pull-right[ ```r summary(lm(y~x1,df)) ``` ``` ## ## Call: ## lm(formula = y ~ x1, data = df) ## ## Residuals: ## Min 1Q Median 3Q Max ## -4.642 -2.308 -0.119 1.479 6.423 ## ## Coefficients: ## Estimate Std. Error t value Pr(>|t|) ## (Intercept) 22.606 0.918 24.62 <2e-16 *** ## x1horse -1.914 1.299 -1.47 0.149 ## x1cat -3.236 1.299 -2.49 0.017 * ## x1dog -0.342 1.299 -0.26 0.794 ## --- ## Signif. codes: 0 '***' 0.001 '**' 0.01 '*' 0.05 '.' 0.1 ' ' 1 ## ## Residual standard error: 2.9 on 36 degrees of freedom ## Multiple R-squared: 0.181, Adjusted R-squared: 0.113 *## F-statistic: 2.65 on 3 and 36 DF, p-value: 0.0633 ``` ] --- count:false class: extra exclude: FALSE # partitioning variance <img src="jk_img_sandbox/anova.png" width="1288" /> .footnote[ The terminology here can be a nightmare. `\(SS_{between}\)` sometimes gets referred to as `\(SS_{model}\)`, `\(SS_{condition}\)`, `\(SS_{regression}\)`, or `\(SS_{treatment}\)`. Meanwhile `\(SS_{residual}\)` also gets termed `\(SS_{error}\)`. To make it all worse, there are inconsistencies in the acronyms used, `\(SSR\)` vs. `\(RSS\)`! ] --- count:false class: extra exclude: FALSE # more anova .pull-left[ with multiple predictors, sums of squares can be calculated differently 1. Sequential Sums of Squares = Order matters 2. <p style="opacity:0.4">Partially Sequential Sums of Squares</p> 3. <p style="opacity:0.4">Partial Sums of Squares</p> ] .pull-right[ __sequential SS__ ```r anova(lm(y~x1+x2,df)) ``` ``` ## Analysis of Variance Table ## ## Response: y ## Df Sum Sq Mean Sq F value Pr(>F) ## x1 3 67.1 22.4 12.7 1e-05 *** ## x2 2 243.7 121.9 69.2 1e-12 *** ## Residuals 34 59.9 1.8 ## --- ## Signif. codes: 0 '***' 0.001 '**' 0.01 '*' 0.05 '.' 0.1 ' ' 1 ``` ```r anova(lm(y~x2+x1,df)) ``` ``` ## Analysis of Variance Table ## ## Response: y ## Df Sum Sq Mean Sq F value Pr(>F) ## x2 2 225.4 112.7 64.0 3.0e-12 *** ## x1 3 85.5 28.5 16.2 1.1e-06 *** ## Residuals 34 59.9 1.8 ## --- ## Signif. codes: 0 '***' 0.001 '**' 0.01 '*' 0.05 '.' 0.1 ' ' 1 ``` ] --- count:false class: extra exclude: FALSE # more anova .pull-left[ with multiple predictors, sums of squares can be calculated differently 1. <p style="opacity:0.4">Sequential Sums of Squares</p> 2. <p style="opacity:0.4">Partially Sequential Sums of Squares</p> 3. Partial Sums of Squares = Each one calculated as if its the last one in sequential SS (order doesn't matter). ] .pull-right[ __partial SS__ ```r car::Anova(lm(y~x1+x2,df), type="III") ``` ``` ## Anova Table (Type III tests) ## ## Response: y ## Sum Sq Df F value Pr(>F) ## (Intercept) 3869 1 2196.1 < 2e-16 *** ## x1 85 3 16.2 1.1e-06 *** ## x2 244 2 69.2 1.0e-12 *** ## Residuals 60 34 ## --- ## Signif. codes: 0 '***' 0.001 '**' 0.01 '*' 0.05 '.' 0.1 ' ' 1 ``` ```r car::Anova(lm(y~x2+x1,df), type="III") ``` ``` ## Anova Table (Type III tests) ## ## Response: y ## Sum Sq Df F value Pr(>F) ## (Intercept) 3869 1 2196.1 < 2e-16 *** ## x2 244 2 69.2 1.0e-12 *** ## x1 85 3 16.2 1.1e-06 *** ## Residuals 60 34 ## --- ## Signif. codes: 0 '***' 0.001 '**' 0.01 '*' 0.05 '.' 0.1 ' ' 1 ``` ] --- class: extra exclude: FALSE count:false # Why use lm vs ANOVA? ANOVA requires post-hoc test to compare specific differences, but it has the advantage of conducting fewer tests (initially). - ANOVA asks the question: - "*are there differences* between group means? - "*is there an effect* of x?" - "*does* x predict y?" - the coefficient tests from the linear model ask - "*what are the differences* between group means? - "*what is the effect* of x?" - "*how does* x predict y?" --- count:false class: extra exclude: FALSE # anova() as model comparison .pull-left[ because ANOVA provides test of __set of parameters being simultaneously zero__, we can use this for model comparison. e.g. - "does the addition of `\(\textrm{<predictor(s)>}\)` improve our model of y?" ```r m0<-lm(y~1, df) m1<-lm(y~x1, df) m2<-lm(y~x1+x2, df) m3<-lm(y~x1+x2+x3, df) ``` ] .pull-right[ `anova()` function to compare models. ```r anova(m1, m2) ``` ``` ## Analysis of Variance Table ## ## Model 1: y ~ x1 ## Model 2: y ~ x1 + x2 ## Res.Df RSS Df Sum of Sq F Pr(>F) ## 1 36 303.6 ## 2 34 59.9 2 244 69.2 1e-12 *** ## --- ## Signif. codes: 0 '***' 0.001 '**' 0.01 '*' 0.05 '.' 0.1 ' ' 1 ``` ```r anova(m1, m3) ``` ``` ## Analysis of Variance Table ## ## Model 1: y ~ x1 ## Model 2: y ~ x1 + x2 + x3 ## Res.Df RSS Df Sum of Sq F Pr(>F) ## 1 36 303.6 ## 2 33 59.9 3 244 44.8 9.9e-12 *** ## --- ## Signif. codes: 0 '***' 0.001 '**' 0.01 '*' 0.05 '.' 0.1 ' ' 1 ``` ] --- count:false class: extra exclude: FALSE # Partitioning - ANOVA <img src="jk_img_sandbox/anova.png" width="1288" /> --- count:false exclude: FALSE # Partitioning - ANOVA (Rpt Measures) <img src="jk_img_sandbox/rmanova.png" width="1288" /> --- count:false class: extra exclude: FALSE # Rpt Measures ANOVA in R .pull-left[ 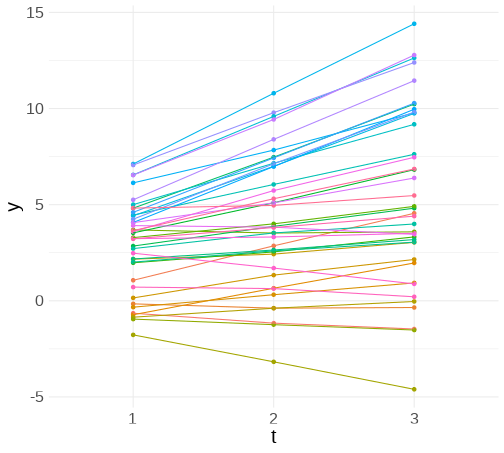<!-- --> ] .pull-right[ ```r library(ez) ezANOVA(data = df, dv = y, wid = subject, within = t) ``` ``` ## $ANOVA ## Effect DFn DFd F p p<.05 ges ## 2 t 2 78 36.7 5.99e-12 * 0.0738 ## ## $`Mauchly's Test for Sphericity` ## Effect W p p<.05 ## 2 t 0.00899 1.34e-39 * ## ## $`Sphericity Corrections` ## Effect GGe p[GG] p[GG]<.05 HFe p[HF] p[HF]<.05 ## 2 t 0.502 4.14e-07 * 0.502 4.12e-07 * ``` ] --- count:false class: extra exclude: FALSE # Mixed ANOVA in R .pull-left[ 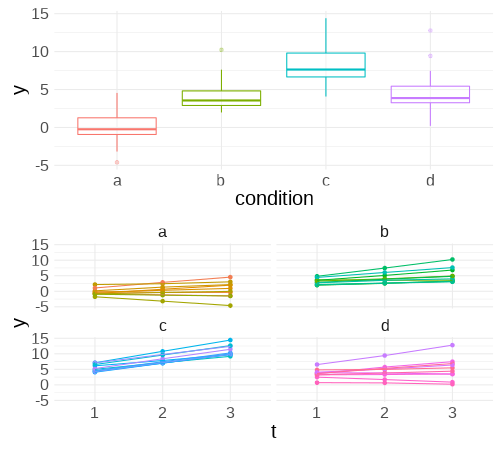<!-- --> ] .pull-right[ ```r library(ez) ezANOVA(data = df, dv = y, wid = subject, within = t, between = condition) ``` ``` ## $ANOVA ## Effect DFn DFd F p p<.05 ges ## 2 condition 3 36 31.5 3.65e-10 * 0.695 ## 3 t 2 72 74.2 3.24e-18 * 0.215 ## 4 condition:t 6 72 14.3 1.16e-10 * 0.137 ## ## $`Mauchly's Test for Sphericity` ## Effect W p p<.05 ## 3 t 0.0195 1.16e-30 * ## 4 condition:t 0.0195 1.16e-30 * ## ## $`Sphericity Corrections` ## Effect GGe p[GG] p[GG]<.05 HFe p[HF] p[HF]<.05 ## 3 t 0.505 2.39e-10 * 0.505 2.35e-10 * ## 4 condition:t 0.505 2.43e-06 * 0.505 2.41e-06 * ``` ] --- count:false exclude: FALSE class: extra, inverse, center, middle, animated, rotateInDownLeft # End